
- Remove audio background noise twistedwave software#
- Remove audio background noise twistedwave Bluetooth#
The way to tell is to just run one of the example files on an Arduino with nothing else going on. Linear predictive coding - Wikipediaīy its nature it will produce audio artifacts, which is what you might be thinking is background noise. This uses a system known as LPC ( Linear predictive Encoding ) which is an ultra compression technique like an early MP3.
Remove audio background noise twistedwave software#
OK now the key thing that your software tells me is that you are using the Talkie library to generate your sounds.
Remove audio background noise twistedwave Bluetooth#
Result2 = stretchĬv2.imwrite("dark_image_stretch.jpg", stretch)Ĭv2.imwrite("dark_image_morph.jpg", morph)Ĭv2.imwrite("dark_image_threshold.jpg", thresh)Ĭv2.imwrite("dark_image_result1.jpg", result1)Ĭv2.imwrite("dark_image_result2.I have used a Lightware distance sensor and Wireless Digital Bluetooth Audio Transmitter(Amazon) Thresh = cv2.threshold(gray, 0, 255, cv2.THRESH_BINARY+cv2.THRESH_OTSU)Ĭontours = cv2.findContours(thresh, cv2.RETR_EXTERNAL, cv2.CHAIN_APPROX_NONE)Ĭontours = contours if len(contours) = 2 else contoursīig_contour = max(contours, key=cv2.contourArea)Ĭv2.drawContours(result1,, 0, (255,255,255), 1)Ĭontour = np.zeros((stretch.shape), dtype=np.uint8)Ĭv2.drawContours(contour,, 0, 255, -1) #thresh = cv2.threshold(gray, 30, 255, cv2.THRESH_BINARY)\ Gray = cv2.cvtColor(morph,cv2.COLOR_BGR2GRAY) Morph = cv2.morphologyEx(morph, cv2.MORPH_OPEN, kernel) Kernel = cv2.getStructuringElement(cv2.MORPH_ELLIPSE, (11,11)) Morph = cv2.morphologyEx(stretch, cv2.MORPH_CLOSE, kernel)
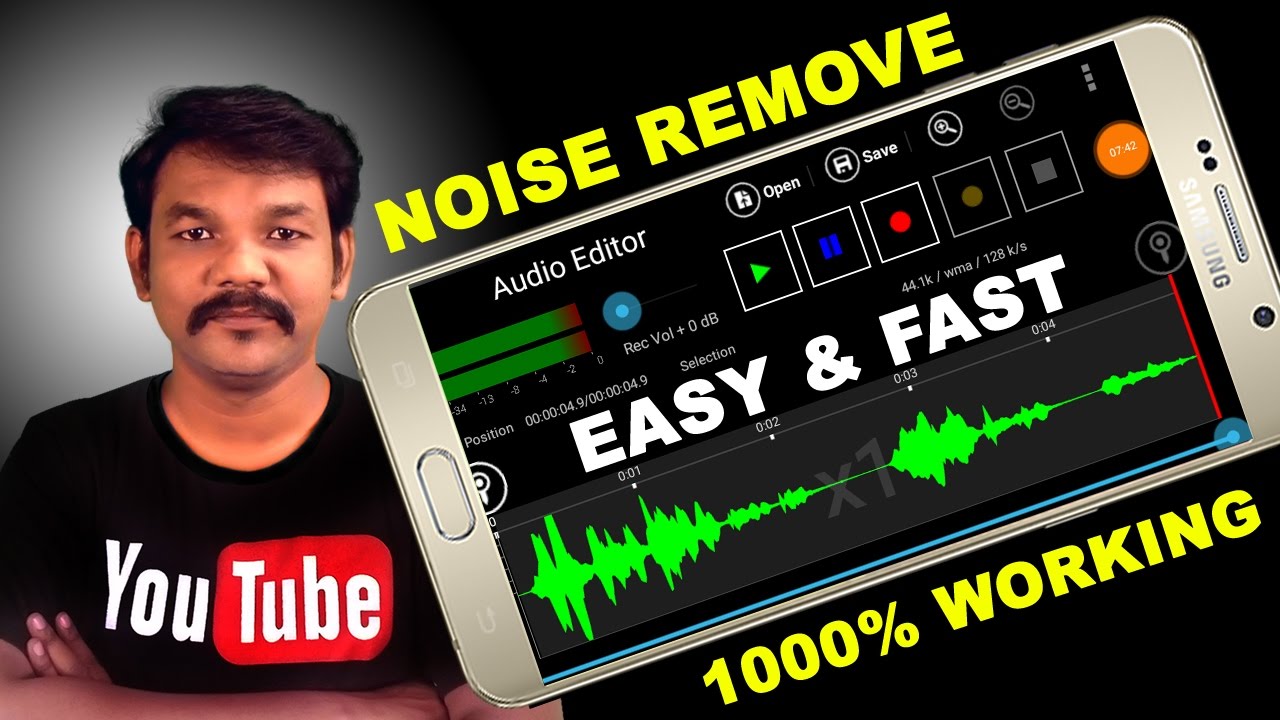

Kernel = cv2.getStructuringElement(cv2.MORPH_ELLIPSE, (101,101)) Stretch = _intensity(img, in_range='image', out_range=(0,255)) Extract the contour region from the stretched image and place it on a black background Draw the contour outline on the stretched image Here is one way in Python/OpenCV/Skimage - Read the input Thank you very much for any hint you can give me! So in short, I would like to remove the background noise, so that only the information i'm interested in stays in the image.īackground noise can very a lot, and I have images where I know that only the background noise is in there and nothing else. :) But besides that, I would like to know if you guys think this is the right direction for this kind of problem? The dominant color should be the background noise.Īnd then subtract this dominant color from the images who has clusters in it: def substract(image, dominant): So my idea was to find the dominant color in this negative control: from skimage import ioĬolors, count = np.unique(a.reshape(-1, a.shape), axis=0, return_counts=True) Everything in there is just the background-noise. I have images where I know, that there are no clusters in it (negative control).
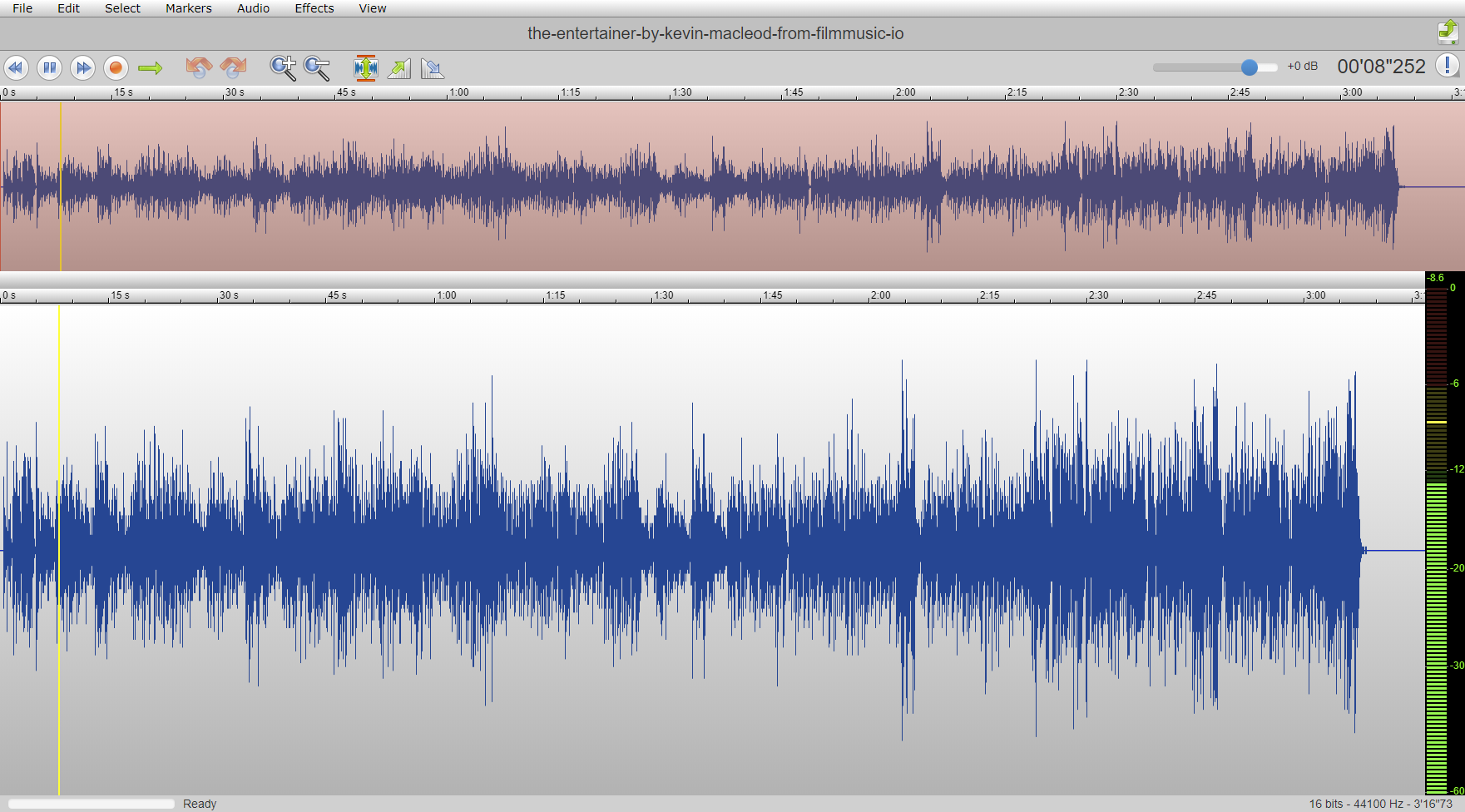
The background noise can very a lot! The image above is a sample, where there is not that much background noise, but it can be a lot worse. Then I could increase the brightness of the image and (I think) it should be easier to identify those clusters. So what I would like to do now is remove the background noise, so that only the clusters are left. With images like this, where the cluster is very dark or images where the background noise is very strong and looks very similar to the actual cluster, i have problems detecting them. I use cv2.findContours for this and this already works very good for images where this clusters have a good brightness and the background noise is not too strong.

I want to find those clusters and count them. In the center there is a cluster (hard to see). I'm new to OpenCV and tried multiple things but still have some problems.
